In an increasingly interconnected global economy, the ability to accurately forecast recessions has become a critical focus for economists and analysts. Economic forecasts serve as essential tools for understanding potential downturns, guiding policy decisions, and informing investment strategies. However, the reliability of these forecasts in predicting global recessions has come under scrutiny, particularly in light of recent economic events that have challenged traditional forecasting models.
The complexity of economic systems, influenced by a myriad of factors such as geopolitical tensions, technological advancements, and unprecedented monetary policies, complicates the forecasting landscape. For instance, the recent use of asset purchase programs (APPs) by central banks has altered the dynamics of the yield curve, a historically reliable indicator of recession probabilities. This shift raises questions about the effectiveness of conventional forecasting methods, which often rely heavily on interest rate differentials and other traditional economic indicators.
Moreover, the advent of machine learning and advanced data analytics has introduced new methodologies for predicting economic cycles. These innovative approaches aim to enhance the accuracy of forecasts by incorporating vast datasets and identifying patterns that may not be evident through traditional analysis. Studies have shown that machine learning models can outperform traditional econometric models in predicting business cycle phases, including recessions, by leveraging real-time data and complex algorithms.
As we delve into the reliability of economic forecasts in predicting global recessions, it is essential to examine the methodologies employed, the historical accuracy of these forecasts, and the evolving landscape of economic indicators. By understanding the strengths and limitations of current forecasting practices, economists and analysts can better navigate the uncertainties of the global economy and make informed decisions that mitigate risks associated with potential recessions.
Methodologies for Economic Forecasting
Economic forecasting involves various methodologies that aim to predict future economic conditions, including recessions. Understanding these methodologies is crucial for evaluating their reliability. Below are some of the primary approaches used in economic forecasting:
Econometric Models
Econometric models use statistical techniques to analyze economic data and establish relationships between different economic variables. These models often rely on historical data to predict future trends. Common econometric models include:
- Time Series Analysis: This method analyzes historical data points collected over time to identify trends and seasonal patterns. It is often used to forecast GDP growth and unemployment rates.
- Structural Models: These models incorporate economic theory to explain relationships between variables. They can be complex and require significant data inputs, making them challenging to implement accurately.
While econometric models have been widely used, their reliability has been questioned, especially in predicting turning points like recessions. Studies have shown that forecasters often fail to predict recessions until they are already underway, indicating a lag in the responsiveness of these models to changing economic conditions
Leading Economic Indicators (LEIs
Leading economic indicators are statistics that tend to change before the economy as a whole changes. Analysts often use these indicators to predict future economic activity. Some commonly used LEIs include:
- Stock Market Performance: A rising stock market can indicate investor confidence and potential economic growth.
- Manufacturing Activity: Increases in manufacturing orders can signal future economic expansion.
- Consumer Confidence Index: Higher consumer confidence typically leads to increased spending, which can drive economic growth.
While LEIs can provide valuable insights, they are not foolproof. For example, the stock market can be influenced by factors unrelated to the economy, leading to misleading signals about future economic conditions.
You can also Read: Why Consumer Spending Is a Key Economic Indicator

Machine Learning and Big Data Analytics
The rise of machine learning and big data analytics has transformed economic forecasting. These methodologies leverage vast amounts of data to identify patterns and make predictions. Key features include:
- Real-Time Data Processing: Machine learning models can analyze data as it becomes available, allowing for more timely forecasts.
- Pattern Recognition: Advanced algorithms can detect complex relationships between variables that traditional models might overlook.
Research indicates that machine learning models can outperform traditional econometric models in predicting economic downturns, as they can adapt to new information more rapidly and effectively
Qualitative Forecasting
Qualitative forecasting relies on expert judgment and insights rather than quantitative data. This approach is particularly useful in situations where data is scarce or unreliable. Techniques include:
- Delphi Method: A structured process that gathers expert opinions through multiple rounds of questioning to reach a consensus forecast.
- Focus Groups: Engaging groups of experts or stakeholders to discuss and predict economic trends based on their experiences and insights.
Evaluating the Reliability of Economic Forecasts
Evaluating the reliability of economic forecasts, particularly in predicting global recessions, involves analyzing several key factors. These factors include the accuracy of past forecasts, the methodologies used, and the inherent uncertainties in economic data. Below are the primary considerations for assessing the reliability of economic forecasts:
Historical Accuracy of Forecasts
Understanding how accurate past forecasts have been is crucial for evaluating their reliability. Key points include:
- Track Record of Predictions: Historical data shows that many economic forecasts have failed to predict recessions accurately. For example, during the 2008 financial crisis, most forecasters did not anticipate the downturn until it was already underway.
- Consensus Forecasts: Consensus forecasts, which aggregate predictions from multiple sources, often provide a more balanced view. However, they can still be subject to collective biases and may not always reflect the most accurate predictions.
Methodological Rigor
The methodologies employed in creating economic forecasts significantly impact their reliability. Important aspects to consider include:
Model Selection: The choice of econometric models (e.g., time series analysis, structural models) can influence forecast outcomes. Models that incorporate a wide range of variables and historical data tend to produce more reliable forecasts.
Data Quality: The accuracy of forecasts is heavily dependent on the quality of the data used. Incomplete or outdated data can lead to misleading predictions. Analysts must ensure they are using the most current and comprehensive datasets available.
Learn More:
Enough With the Economic Doomsaying | TIME
Why Energy Investment Is Crucial for Growth in Emerging Markets
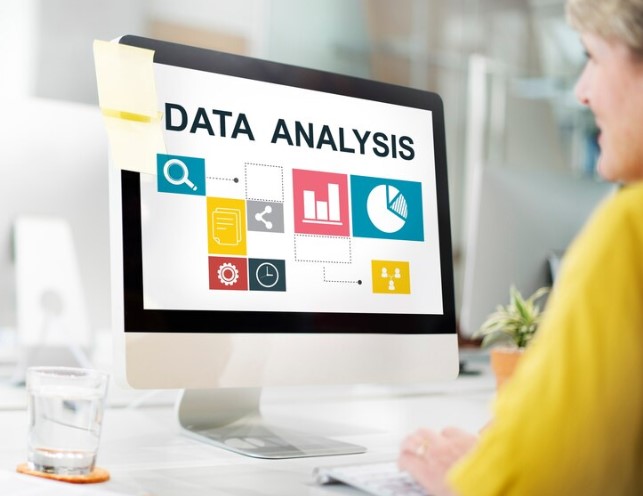
Incorporating Uncertainty
Economic forecasting is inherently uncertain due to the complexity of economic systems. Key considerations include:
- Risk Assessment: Forecasters must account for various risks, including geopolitical events, natural disasters, and changes in consumer behavior, which can all impact economic conditions unexpectedly.
- Scenario Analysis: Utilizing scenario analysis allows forecasters to explore different potential outcomes based on varying assumptions. This approach can help in understanding the range of possible economic futures and the associated risks.
Use of Advanced Techniques
The integration of advanced forecasting techniques can enhance reliability:
Machine Learning Models: These models can analyze vast datasets and identify patterns that traditional methods may overlook. Research indicates that machine learning can improve the accuracy of recession predictions by adapting to new information more effectively.
Real-Time Data Analysis: The ability to process and analyze data in real-time allows forecasters to adjust their predictions based on the latest economic developments, improving the timeliness and relevance of forecasts.
Expert Judgment and Qualitative Insights
In addition to quantitative methods, qualitative insights play a vital role in evaluating forecasts:
Expert Opinions: Engaging with economists and industry experts can provide valuable context and insights that quantitative models may miss. The Delphi method, for example, gathers expert opinions to reach a consensus on future economic conditions.
Focus Groups: Conducting focus groups with stakeholders can help identify emerging trends and potential economic shifts, adding depth to the forecasting process.
Read More on: How Globalization Is Changing the Landscape of Higher Education
Sources of Forecast Errors
Understanding the sources of forecast errors is essential for evaluating the reliability of economic forecasts, particularly in predicting global recessions. Various factors contribute to inaccuracies in economic predictions, and recognizing these can help economists and analysts refine their forecasting methods. Below are the primary sources of forecast errors:
Turning Points in the Business Cycle
Forecasting models often struggle to predict turning points in the business cycle, such as the onset of a recession. These turning points can be influenced by sudden changes in economic conditions that are difficult to anticipate. For instance, unexpected shocks, such as financial crises or geopolitical events, can lead to abrupt shifts in economic activity that models fail to capture.
Changes in Labor Productivity Trends
Labor productivity is a critical variable in economic forecasting. Changes in productivity trends can significantly impact economic growth and employment rates. If forecasters do not accurately account for shifts in productivity, their predictions may be overly optimistic or pessimistic, leading to significant errors in forecasting recessions.
Fluctuations in Commodity Prices
Commodity prices, particularly oil, can have a profound effect on the economy. Sudden spikes or drops in prices can influence inflation rates and consumer spending, which are crucial for economic forecasts. For example, a sharp increase in oil prices can lead to higher transportation costs, affecting overall economic activity and potentially triggering a recession.
Data Revisions
Economic data is often subject to revisions as new information becomes available. These revisions can lead to significant changes in the understanding of economic conditions. Forecasts based on preliminary data may not accurately reflect the final, revised figures, resulting in errors. Analysts must be cautious about relying too heavily on initial data releases.
Behavioral Factors
Human behavior plays a significant role in economic activity, and forecasting models may not fully account for psychological factors that influence consumer and business decisions. For instance, changes in consumer confidence can lead to shifts in spending patterns that are difficult to predict. Behavioral economics highlights the importance of understanding these factors in improving forecasting accuracy.
Strategies for Enhancing Forecast Reliability
To improve the reliability of economic forecasts, especially in predicting global recessions, economists and analysts can adopt a variety of strategies. These strategies encompass methodological enhancements, the integration of diverse data sources, and the application of advanced analytical techniques. Below are key strategies for enhancing forecast reliability:
Incorporating a Diverse Set of Economic Indicators
Utilizing a broader range of economic indicators can provide a more comprehensive view of potential economic conditions. Rather than relying solely on traditional metrics like GDP or unemployment rates, forecasters should consider:
- Leading Indicators: Incorporating leading indicators, such as manufacturing activity, stock market trends, and consumer confidence, can help predict economic shifts before they occur.
- Lagging Indicators: While these indicators confirm trends after they occur, analyzing them can provide context and enhance understanding of economic cycles.
- Coincident Indicators: These indicators, such as retail sales and industrial production, can help assess current economic conditions and validate forecasts.

Utilizing Ensemble Forecasting Techniques
Ensemble forecasting combines multiple forecasting models to produce a more robust prediction. This technique leverages the strengths of various models while mitigating their individual weaknesses. Key benefits include:
Reduced Uncertainty: By averaging the outputs of different models, ensemble methods can reduce the impact of outliers and improve overall accuracy .
Diverse Perspectives: Ensemble forecasting incorporates different methodologies, which can provide a more nuanced understanding of economic conditions.
Implementing Real-Time Data Analytics
The use of real-time data analytics allows forecasters to respond more quickly to emerging economic trends. This approach involves:
- Continuous Monitoring: Utilizing real-time data from various sources, including financial markets, social media, and news outlets, can help identify shifts in consumer sentiment and economic activity.
- Adaptive Models: Machine learning algorithms can be employed to adjust forecasts based on the latest data, enabling more timely and accurate predictions.
Scenario Planning and Stress Testing
Scenario planning helps forecasters assess the potential impact of various economic conditions by creating different hypothetical scenarios. This strategy includes:
- Stress Testing Models: By simulating extreme economic conditions (e.g., a sudden recession or a financial crisis), analysts can evaluate how their models perform under stress and identify potential weaknesses.
- Exploring Multiple Outcomes: Scenario planning encourages forecasters to consider a range of possible futures, facilitating better preparation for unexpected economic changes.
Enhancing Collaboration and Expert Consultation
Collaboration among economists, analysts, and industry experts can enhance the forecasting process. Strategies include:
Interdisciplinary Teams: Forming teams that include experts from various fields (e.g., finance, sociology, and behavioral economics) can provide diverse perspectives and insights, enriching the forecasting process.
Expert Panels: Consulting panels of experts can help validate forecasts and provide qualitative insights that quantitative models may overlook.
Investing in Training and Development
Continuous education and training can help economists and analysts stay abreast of the latest developments in forecasting methodologies and technologies. This includes:
- Workshops and Courses: Participating in workshops focused on advanced forecasting techniques, data analytics, and machine learning can enhance skills and knowledge.
- Access to Resources: Providing access to the latest research, software tools, and academic literature can empower analysts to utilize the most effective methodologies.

Future Directions in Economic Forecasting
As the economic landscape continues to evolve, the field of economic forecasting must adapt to new challenges and opportunities. Future directions in this field involve the integration of advanced technologies, innovative methodologies, and a greater emphasis on collaboration among diverse disciplines. Below are key trends and developments that are likely to shape the future of economic forecasting.
Also Read: How Globalization Is Transforming the Service Industry
Embracing Big Data and Analytics
The proliferation of big data presents both challenges and opportunities for economic forecasting. The ability to analyze vast amounts of data from various sources can significantly enhance predictive accuracy.
- Data Sources: Future forecasting will increasingly leverage unconventional data sources, such as social media sentiment analysis, web traffic patterns, and mobile phone usage data. These sources can provide real-time insights into consumer behavior and economic activity.
- Advanced Analytical Tools: The adoption of sophisticated analytics tools, including machine learning and artificial intelligence, will allow economists to identify patterns and correlations that traditional models may miss. This shift toward data-driven forecasting can lead to more timely and accurate predictions.
Enhancing Model Flexibility
The complexity and volatility of modern economies require forecasting models that can quickly adapt to changing conditions.
- Dynamic Models: Future models will likely incorporate dynamic elements that adjust in real time based on new data inputs. This adaptability can improve responsiveness to sudden economic shifts, such as unexpected geopolitical events or natural disasters.
- Integrative Approaches: Combining multiple modeling techniques (e.g., econometric models with machine learning algorithms) can create more robust forecasting frameworks. This integrative approach can leverage the strengths of different methodologies while compensating for their weaknesses.
Conclusion
The complexity of global economies and the inherent uncertainties in forecasting present significant challenges for economists and analysts. As explored throughout this article, the reliability of economic forecasts, particularly in predicting recessions, is influenced by a multitude of factors, including the methodologies employed, the data utilized, and the external conditions surrounding economic activity.
To navigate the complexities of an ever-changing economic landscape, economists and analysts must remain vigilant and adaptable. Continuous education, investment in new technologies, and a commitment to interdisciplinary collaboration will be essential for improving forecasting practices. By embracing these principles, the field of economic forecasting can evolve to meet the demands of policy-makers, businesses, and the general public in understanding and preparing for future economic conditions.
In conclusion, while forecasting global recessions presents significant challenges, the integration of innovative methodologies, a focus on behavioral insights, and a commitment to transparency can enhance the reliability of predictions. As we move forward, it is vital for economists and analysts to leverage these strategies to better anticipate and respond to the dynamic nature of the global economy.